Artificial intelligence has become a cornerstone of technological advancements, revolutionizing industries and transforming the way we interact with technology. Two terms that often arise in discussions about AI are “Deep Learning” and “Machine Learning.” While they are both subfields of AI, they have distinct characteristics and applications. In this article, we will delve into the nuances of Deep Learning and Machine Learning, examine their differences, explore real-world applications, and understand their impact on various industries.
Deep Learning Vs Machine Learning
Deep Learning and Machine Learning are integral components of artificial intelligence. They differ in their approaches, algorithms, and capabilities. Let’s explore the key distinctions between these two domains.
What is Deep Learning?
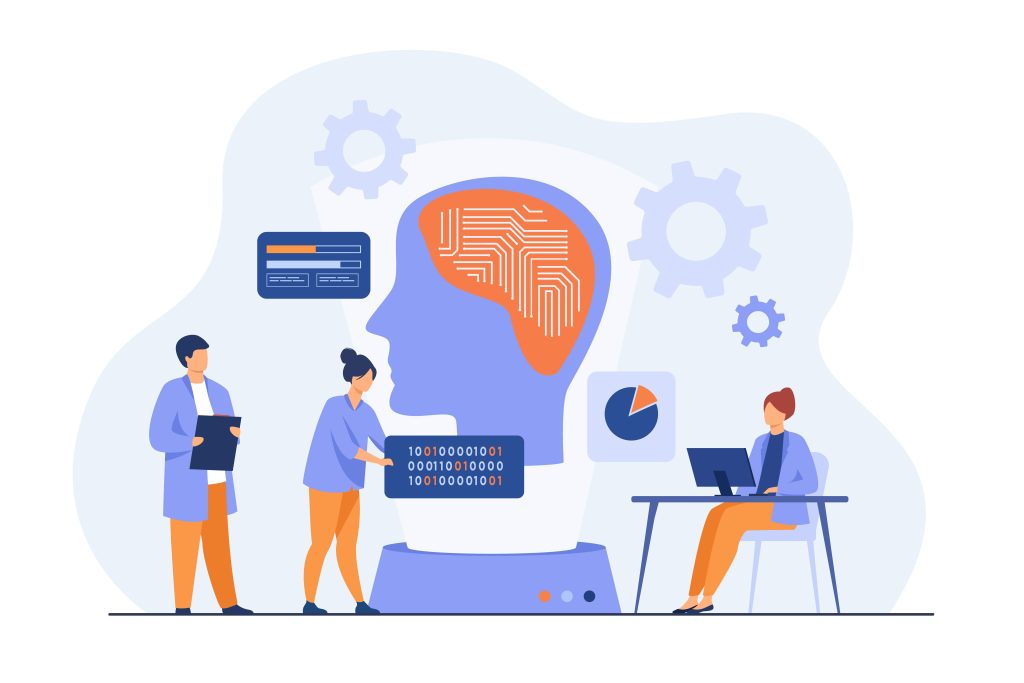
Deep Learning, as the name suggests, involves training artificial neural networks to learn and understand data representations by recognizing intricate patterns and hierarchies. It mimics the structure and functionality of the human brain, consisting of interconnected layers of artificial neurons called artificial neural networks (ANNs).
How Deep Learning Works?
Deep Learning algorithms employ multiple layers of interconnected nodes, where each node processes and transforms data using weighted connections. These connections, also known as synapses, help in determining the importance of each node’s output for subsequent layers.
Advantages of Deep Learning
1. Uncovering Complex Patterns
Deep Learning models excel at recognizing intricate patterns and relationships in data, enabling more accurate predictions and analysis.
2. Handling Large-Scale Data
Deep Learning algorithms can efficiently process vast amounts of data, making them ideal for applications such as image and speech recognition, natural language processing, and autonomous vehicles.
3. Feature Extraction
Deep Learning models automatically learn relevant features from raw data, eliminating the need for manual feature engineering.
What Does Mean By Machine Learning?
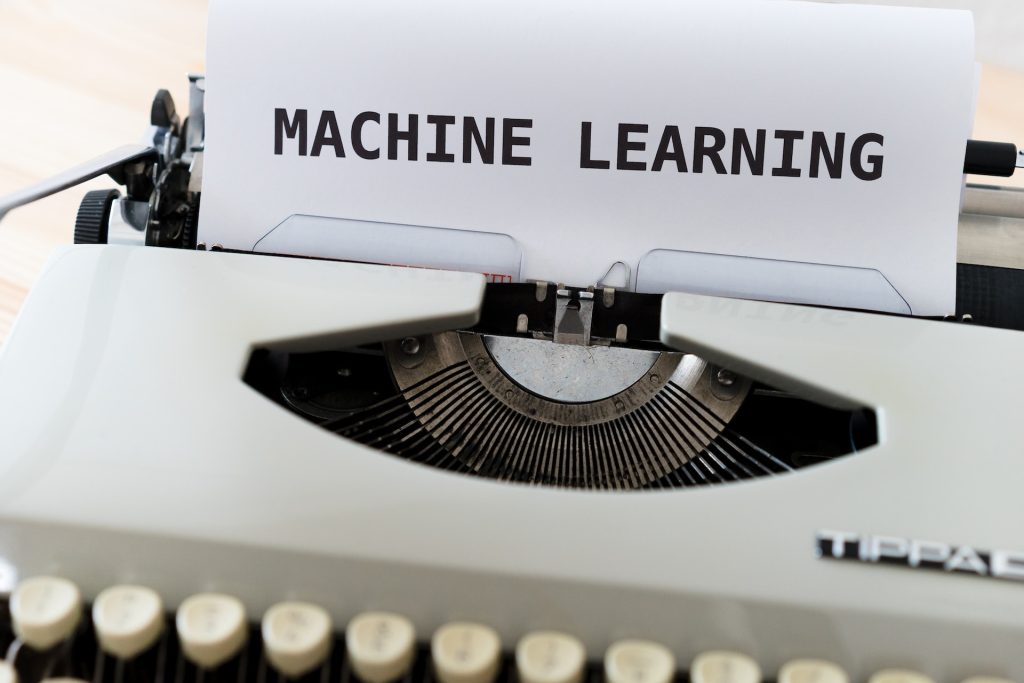
Machine Learning, on the other hand, focuses on developing algorithms that allow computers to learn from and make predictions or decisions based on data. It encompasses a broad range of techniques that aim to find patterns and statistical relationships within datasets.
How Machine Learning Works?
Machine Learning algorithms are trained on historical data to recognize patterns, extract insights, and make informed predictions or decisions. They rely on statistical models and optimization techniques to create algorithms that improve over time as more data becomes available.
Advantages of Machine Learning
1. Flexible and Customizable
Machine Learning algorithms can be adapted to various problem domains, making them versatile and widely applicable.
2. Interpretability
Machine Learning models often provide interpretable results, allowing stakeholders to understand the factors influencing predictions or decisions.
3. Efficient Resource Utilization
Machine Learning algorithms can handle large datasets effectively, optimizing resource usage for scalability.
Deep Learning Vs Machine Learning: Applications in Real-World Scenarios
Both Deep Learning and Machine Learning find applications across various industries, facilitating advancements and driving innovation. Let’s explore some practical use cases where each approach excels.
Deep Learning Applications
1. Image and Object Recognition
Deep Learning has revolutionized image recognition tasks, enabling machines to identify objects, scenes, and patterns with remarkable accuracy. This technology powers facial recognition systems, self-driving cars, and content-based image retrieval.
2. Natural Language Processing
Deep Learning algorithms have transformed the field of Natural Language Processing (NLP). They facilitate language translation, sentiment analysis, chatbots, and voice assistants, improving human-machine interaction.
3. Medical Diagnostics
In healthcare, Deep Learning plays a vital role in medical imaging analysis, disease diagnosis, and personalized treatment plans. Its ability to analyze complex medical images assists radiologists and enhances patient care.
Machine Learning Applications
1. Fraud Detection
Machine Learning algorithms can detect fraudulent activities by analyzing patterns and anomalies in transactional data. They enable financial institutions to identify suspicious behavior, preventing fraudulent transactions.
2. Customer Relationship Management
Machine Learning techniques enhance customer relationship management by analyzing customer data, predicting buying behavior, and providing personalized recommendations. This enables businesses to deliver targeted marketing campaigns and improve customer satisfaction.
3. Predictive Maintenance
Machine Learning models can predict equipment failures by analyzing sensor data and historical maintenance records. This proactive approach helps industries optimize maintenance schedules, reduce downtime, and minimize costs.
FAQs (Frequently Asked Questions)
1. What is the difference between Deep Learning and Machine Learning?
Deep Learning focuses on training artificial neural networks to recognize complex patterns and hierarchies, while Machine Learning aims to develop algorithms that enable computers to learn from data and make predictions or decisions.
2. Which approach is more suitable for image recognition tasks?
Deep Learning excels in image recognition tasks due to its ability to uncover intricate patterns and hierarchies, making it an ideal choice for applications like object detection and facial recognition.
3. Can Deep Learning algorithms handle large-scale datasets?
Yes, Deep Learning algorithms can efficiently process large-scale datasets, making them suitable for applications like speech recognition, natural language processing, and autonomous vehicles.
4. Are Machine Learning models interpretable?
Yes, Machine Learning models often provide interpretable results, allowing stakeholders to understand the factors influencing predictions or decisions.
5. How does Deep Learning contribute to medical diagnostics?
Deep Learning is instrumental in medical diagnostics as it can analyze complex medical images, assist in disease diagnosis, and aid in personalized treatment plans.
6. What is the primary advantage of Machine Learning algorithms?
A: One of the primary advantages of Machine Learning algorithms is their flexibility and adaptability to various problem domains, making them widely applicable.
Conclusion
Deep Learning and Machine Learning are two integral pillars of artificial intelligence, each with its own unique characteristics and applications. Deep Learning excels in uncovering complex patterns and hierarchies, making it ideal for image and speech recognition, natural language processing, and medical diagnostics. On the other hand, Machine Learning enables data-driven predictions and decisions, finding applications in fraud detection, customer relationship management, and predictive maintenance. Understanding the distinctions between Deep Learning and Machine Learning empowers us to harness their potential and unlock the true capabilities of AI.